Selection For Feed Efficiency In Beef Cattle
The Australian Beef Co-operative Research Centre is currently mapping genes for net feed intake using high-density single nucleotide polymorphisms (SNPs). The research is published in Volume 18 of Recent Advances in Animal Nutrition - Australia.Summary
The feed conversion efficiency of a beef herd can be increased by increasing net reproductive rate, by increasing growth rate without increasing cow maintenance requirements or by decreasing net feed intake (NFI). Although NFI is heritable and responds to selection, the high cost of measuring feed intake on individual cattle has prevented cattle breeders from measuring this trait and using the measurements for selection. Many physiological processes contribute to variation in NFI and perhaps hundreds or even thousands of genes explain its heritability. The Australian Beef Co-operative Research Centre is currently mapping genes for NFI using high-density single nucleotide polymorphisms (SNPs). The aim of this work is to identify genes responsible for variation in NFI and to develop a prediction equation that estimate breeding value for NFI from a panel of SNP genotypes covering the whole genome. The prediction equation will be utilised to calculate estimated breeding values (EBVs) for NFI within Breedplan and these will be combined with EBVs for other traits to select for overall profitability.
Introduction
The cost of feed accounts for at least 60 per cent of the variable costs of beef production systems (Grifith et al., 2004). This cost applies to cattle at pasture and to feedlot cattle, but the cost is sometimes underestimated in the case of cattle at pasture. The high cost is apparent when a reduction in feed requirement per animal is considered equivalent to an opportunity to increase stocking rate. As most feed is consumed by the cow herd, efforts to reduce feed costs should not focus only on increasing feed conversion efficiency (FCE) in the feedlot. A better objective is to increase the whole herd FCE. In this review, we first define the traits that determine herd FCE. One of these traits is residual or net feed intake (NFI) and we consider the physiological traits that contribute to NFI, the genetic parameters of NFI, attempts to map genes for NFI and methods of selecting for improved NFI.
Herd feed conversion efficiency
Herd FCE may be defined as the beef produced divided by the feed eaten by the herd over a year. The main factors influencing this variable can be understood from a simple model consisting of a single cow and the offspring she produces in one year:
Herd FCE = w o (f–l) /[fc + f × fo]
where w o is slaughter weight, f is weaning rate, l is cow loss rate, f c is feed eaten by the cow and fo is feed eaten by the offspring from birth to slaughter or upon entering the herd.
This equation shows that the herd FCE could be increased by increasing the fertility of the herd (weaning rate) or by decreasing the cow loss rate. The definition of cow loss rate depends on the value of cows at the end of their life. If culled cows have no value, then cow loss rate includes cows that die and cows that are culled. If culled cows are worth as much as a slaughter offspring, then cow loss rate is the cow death rate. The actual value may be intermediate between these two extremes.
Feed eaten by a cow is partitioned between maintenance, lactation and pregnancy. The feed required by a slaughter offspring is its live weight at slaughter minus birth weight multiplied by its food conversion ratio (FCR; i.e., 1/FCE). Slaughter age may be determined by a management decision and this directly affects slaughter weight and FCR. To maximise herd FCE, there is an optimum age at slaughter when the other parameters, such as fertility and genetic merit, are constant. As age at slaughter and hence weight increases, the animal approaches maturity and hence, fatness and FCR increase. This phenomenon favours a low age at slaughter but is balanced by the constant overhead of cow feed requirement, which must be offset by lower slaughter weight if age at slaughter is reduced. The optimum age at slaughter may depend on mature size, among other factors. The optimum age at slaughter is usually later for cattle of larger mature size. In practice, the economically optimum age for slaughter may be a compromise between the optimum age for herd FCE and the age at which the value of the slaughter animal per kg is maximised. However, here we assume that animals are slaughtered at the optimum age for herd FCE. Under this assumption, herd FCE is almost unaffected by mature size because cattle of large mature size will be slaughtered at heavier weights than cattle of smaller mature size, but this will only compensate for the greater feed requirement and possibly slower reproductive rate of the cows.
What genetic parameters affect herd FCE? As mentioned above, increasing net reproductive rate (f–l) would increase herd FCE, but we will not consider this possibility further. The feed requirement of the slaughter offspring and cow can be expressed as follows:
f o = expected (fo) + ro
f c = expected (fc) + rc
where expected (fo) is the average feed requirement of animals of a defined weight and age, ro is net feed intake of the offspring, expected (fc) is the expected feed requirement of a cow of given weight and milk yield, and r c is net feed intake of the cow.
Herd FCE would be increased by increasing the slaughter weight of the offspring without increasing the cow weight or the feed required for milk production or the net feed intake of either cow or offspring. It may be possible to do this using a crossbreeding system in which a small breed of cow is mated to a large terminal sire breed, provided other problems such as dystocia can be avoided. Cattle that are adapted to their environment, for instance, cattle in tropical Australia that are resistant to ticks and worms, should grow to slaughter weight more quickly than unadapted animals without a proportional increase in cow weight and would thus result in an increase in herd FCE. Conversely, if Brahman cattle are poorly adapted to a feedlot environment and grow slowly with a high FCR, this will depress the herd FCE, of which the feedlot phase is a part. It may also be possible to achieve an increase in growth rate without increasing mature cow size by selecting for a faster-maturing animal, but there are indications that this would increase maintenance requirement and hence NFI.
Reduction of the NFI of the cow or the offspring would increase herd FCE. Conversely, increasing FCE during part of the production cycle may not increase herd FCE. For instance, cattle of large mature size have an elevated FCE during growth between fixed weight endpoints because, at a fixed weight, they are less mature and are depositing less fat. However, because the cows will also be larger and have larger maintenance requirements, herd FCE may not change.
Components of net feed intake
Herd and Arthur (2009) reviewed the physiological basis of variation in NFI. They concluded that although differences in digestibility and body composition contribute to variation in NFI, the largest source of variation is in maintenance requirements and this in turn is due to multiple physiological processes, including the rate of protein turnover, activity, response to stress and possibly mitochondrial efficiency, ion transport and other processes of metabolism. The effect of body composition on NFI is unclear. As fat is energetically expensive to deposit but is cheap to maintain, increased fatness might be associated with increased NFI, as in cattle (Richardson et al., 2001), or decreased NFI, as in poultry and mice (Tixier-Boichard et al., 2002). Metabolic processes in the liver are probably important for feed efficiency. Chen et al. (2011) identified 128 genes in the liver that differed in expression between high and low NFI lines of cattle. The roles these genes play in NFI is still uncertain but there are some fascinating hints at the underlying biology. One network of differentially expressed genes was centred on platelet-derived growth factor and included insulin-like growth factor binding protein 3 (IGFBP3). IGFBP3 influences the availability of IGF1 and serum concentrations of IGF1 are correlated with NFI as reviewed below. IGFBP3 also influences glucose uptake by adipocytes and fatness is also correlated with NFI (see review below).
Genetic variation in net feed intake
A difference between Bos indicus and Bos taurus cattle in fasting metabolic rate was recognised some time ago by Vercoe and Frisch (1977). The lower fasting metabolic rate of Bos indicus would be expected to lower their maintenance requirement and hence NFI. Although they were not designed as breed comparisons and the cattle were not managed together from birth, the studies of Robinson and Oddy (2004) and Barwick et al. (2009) did detect the expected differences between breeds in NFI. These studies showed lower NFI in tropical breeds than in temperate breeds (Robinson and Oddy, 2004) and lower NFI in Brahmans than in composite breeds such as the Santa Gertrudis (Barwick et al., 2004). However, Schutt et al. (2009) did not observe a lower NFI in Brahmans than in Brahman crossbred cattle. Dairy breeds have also been reported to have a higher maintenance requirement than beef breeds and this should result in a higher NFI.
Within-breed genetic variation in NFI is evidenced by estimates of heritability of approximately 0.25 (Arthur and Herd, 2008) to 0.45 (Crowley et al., 2010). At Trangie experimental station, selection based on NFI in growing cattle produced high and low NFI lines that differed in NFI by 10 per cent (Arthur and Herd, 2008). Differences between the lines in NFI occurred both at pasture and in a feedlot. When feed quality was low, the cattle from the low NFI line had a faster growth rate despite eating less than the cattle from the high NFI line. NFI has been measured in cattle between weaning and 12 months of age (NFI-P) and during finishing in a feedlot (NFI-F). The genetic correlation between these two traits was 0.65 (Jeyaruban et al., 2009). That is, cattle that have a genetically low NFI post weaning tend to have a low NFI in the feedlot, but there is some re-ranking. There also appears to be a positive genetic correlation between NFI in growing cattle and cows because the cows from the high NFI line had a higher NFI than the cows from the low NFI line (Archer et al., 2002).
NFI is genetically positively correlated with subcutaneous and intramuscular fat content (Jeyaruban et al., 2009). This is expected because fat contains more energy than lean, but requires less energy to maintain. Therefore, it is not surprising that the correlation between NFI and fatness varies between species, and even within cattle. Jeyaruban et al. (2009) reported that the genetic correlation is lower for NFI-F than NFI-P and lower in heifers than in steers. Barwick et al. (2010) suggested that the correlation increases as the time between the measurement of NFI and the measurement of fat depth increases. Although NFI was initially reported to be correlated with IGF concentration in blood (Moore et al., 2005), Jeyaruban et al.(2009) found almost no correlation using a larger data set.
Mapping genes for net feed intake
The genes controlling NFI are currently unknown. However, it is possible to map genes for NFI to a location in the genome using linkage disequilibrium (LD) and genetic markers such as single nucleotide polymorphisms (SNPs). LD is a non-random association between the alleles at the gene for NFI and the alleles at the SNP. This generates an association between the alleles at the SNP and NFI. That is, the SNP appears to have an effect on NFI but it is merely in LD with a gene affecting NFI. LD is only strong when the gene and the SNP are closely linked on the chromosome; thus, a SNP associated with NFI indicates a gene for NFI in the vicinity of the SNP. Using SNPs covering the whole genome, it is possible to detect genes for NFI wherever they are located. This type of experiment is called a genome-wide association study (GWAS).
The Beef CRC has conducted GWAS for NFI in several populations. For instance, cattle from the Trangie NFI selection lines have been genotyped using the Affymetrix (Santa Clara, California, USA) 10,000 SNP assay and cattle from CRC I and II have been genotyped using the Illumina (San Diego, California, USA) 50,000 SNP chip. The results show that numerous genes affect NFI. For instance, the GWAS on the Trangie selection lines detected 100 SNPs that were significantly (P < 0.001) associated with NFI and were spread across many chromosomes. Other GWAS also detected SNPs that are significantly associated with NFI (Barendse et al., 2007).
However, none of the associations between SNPs and NFI have been consistently replicated using independent populations of cattle and no genes or mutations that cause variation in NFI has been conclusively identified. There are three reasons for this. Firstly, because so many genes affect NFI, each gene explains a very small proportion of the variance and most experiments do not include a sufficient number of cattle to detect the effect of any one gene. Secondly, as LD within a breed occurs between genes separated by >1 megabase (Mb), the confidence interval for the position of the NFI gene is large and contains many genes. Thirdly, LD between a gene for NFI and a SNP in one population may not necessarily exist in another population; therefore, these effects are not easy to replicate in multiple populations. The last-mentioned problem could be overcome by using a denser panel of SNPs. When SNP are very close together (<10 kb apart), the LD is similar across breeds (deRoos et al., 2009). Therefore, it should be possible to identify SNPs that are consistently associated with a gene for NFI across breeds, within Bos taurus at least. The Beef CRC is currently genotyping cattle with an 700,000 SNP chip. To overcome the first problem (i.e., the small effect of individual genes on NFI) genotyping using the 700,000 SNP chip will be conducted on a large number of cattle for which NFI data are available. The Beef CRC is currently genotyping 3000 cattle for which NFI records are available. These cattle represent a variety of breeds and crosses but are mainly feedlot steers. Data on cattle of various of stages of life and consuming various feed sources are required to determine whether the genetic markers have consistent effects.
Despite these problems, preliminary results are encouraging. When 2000 cattle from eight breeds were genotyped using a 50,000 SNP chip, 78 SNPs were significantly associated with NFI (P < 0.001; false discovery rate = 64.5 per cent). These 78 SNPs were mapped to 26 bovine chromosomes using the UMD3 genome assembly. Of the 78 SNPs, the 12 most significant were detected on chromosomes 3, 4, 8, 13, 14, 15, 16 and 24 (P ≤ 7.1 × 10–5). Some of these chromosome regions were also found to contain genes for NFI in an independent experiment using the Trangie NFI selection lines genotyped using a 10,000 SNP chip. Figure 1 shows results for chromosome 8 from the 50,000 experiment. There appear to be many genes affecting NFI on this chromosome and in particular, one near 82 Mb and one near 90 Mb. A gene affecting NFI near 90 Mb was also detected in the 10,000 SNP chip experiment. SNPs with a significant effect on NFI near 90 Mb were found in Bos taurus and Bos taurus × Bos indicus cattle but not in Bos indicus cattle, indicating that this gene may only be segregating in Bos taurus (Figure 1).
The region between 90 Mb and 91Mb, where the most significant SNPs are located, contains at least two genes that could potentially influence animal growth or metabolism-related phenotypes. The SNP with the highest F value is within the SHC gene (related to src). SHC is thought to be involved in signalling pathways associated with neurotrophin, chemokine and insulin signalling. A second gene in close proximity to SHC encodes a receptor for S1P, a bioactive lysophospholipid. This G-protein coupled receptor is thought to be involved in production of the cytokine, interleukin-1β.
Figure 1. Minus log transformation of P values for NFI along chromosome 8 in Bos indicus (Bi), Bos taurus (Bt) and composite (Bt × Bi) breed lines, combining all data.
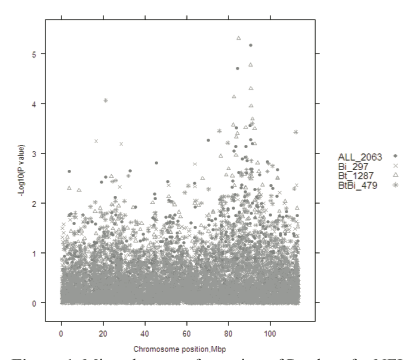
Incorporating net feed intake into a breeding objective
In practice, one would never advocate selection for NFI alone. The aim of selection should be to maximise the profitability of the future herd. A breeding objective is a formula that takes genetic values of traits, such as slaughter weight, as inputs and produces profitability as the output. Breeding objectives for various Australian production systems have been calculated using BreedObject software and are used by Australian breed societies. Breeding objectives often calculate the expected feed intake of cattle based on their weight and growth rate and incorporate the cost of feed in calculating profit. This calculation ignores NFI but it is easy to include it because the rest of the profit calculation is unchanged – one merely has to increase or decrease the feed cost above or below that expected according to the breeding value for NFI. Several NFI traits should be included in the breeding objective, for instance, NFI in growing stock, during feedlot finishing and in cows.
The cost of feed for cattle at pasture may be hard to calculate but a convenient and equivalent method for calculating the economic value of NFI is to change the stocking rate. For instance, if feed intake decreases by 10 per cent, stocking rate increases by 10 per cent. Because the only costs that would be changed by this are those that are proportional to the number of cattle (e.g., veterinary costs), the effect on profit should be large (Archer et al., 2004). Although a 10 per cent increase it sale weight also has a high value, this is offset by increased feed requirements for cows and slaughter stock (Archer et al., 2004).
Selection for net feed intake
Once a breeding objective is chosen, the next step is to calculate the selection index, based on measured traits, that best predicts breeding value for the objective. If it is done correctly, it accounts for all the correlations between traits in the objective and the index. Even if NFI is not measured, including NFI in the objective will improve the accuracy of the selection index because correlations between NFI and measured traits will be accounted for. Barwick et al. (2010) calculated that even without NFI measurement, including NFI in the objective would improve the index as a result of selection for lower fatness, provided fat depth was measured. However, NFI measurement results in greater improvements in the accuracy of the index and greater genetic gain in the objective (i.e., profit). The major problem with NFI measurement of individual bulls is the cost ($A150–450 per bull). The total cost can be reduced by conducting selection of the best bulls in two stages. First, selection is practiced on all other traits and only the best bulls that are selected in this first stage are subjected to NFI measurement in the second stage (Exeter et al., 2000). However, the profitability of NFI measurement is still marginal (Arthur and Herd, 2008). As a stud breeder is unlikely to be able to capture the full value of improved bulls, it is not surprising that very few have invested in NFI measurement.
Adoption of selection for NFI will probably not occur unless a much less expensive method of NFI measurement is developed or a trait closely correlated with NFI and inexpensive to measure is discovered. Initially, it is appeared that IGF concentration in blood was correlated with NFI and the IGF levels of many bulls were measured, but subsequent studies showed that the correlation was weak and of little use (Jeyaruban et al., 2009).
The most likely method of selecting for NFI in the near future is genomic selection. Genomic selection (Meuwissen et al., 2001) refers to the use of a genome-wide panel of dense DNA markers in an equation that predicts breeding value for a particular trait. Genomic selection for milk production traits in dairy cattle is very successful (Hayes et al., 2009) and there is no reason to believe that it could not be successful in beef cattle. However, it is harder to achieve acceptable accuracy in beef cattle than in dairy cattle. Genomic selection requires a large dataset of animals that have been genotyped for the SNP markers and for which the trait has been measured. There are thousands of dairy bulls with EBVs based on accurate progeny tests that have been genotyped using a SNP chip, most of which are Holstein. In beef cattle, especially for NFI, a smaller number of animals have been subjected to measurement and they are spread across many breeds and two subspecies (Bos taurus and Bos indicus). However, by using a denser set of markers and more animals, we expect to obtain a prediction equation with enough accuracy to be useful. Dense markers are necessary because they should provide markers that are close to the causal polymorphisms and hence in the same phase of LD with the causal polymorphism in different breeds (deRoos et al., 2009). These markers will then have an association with NFI that is consistent across breeds.
Conclusion
The aim of genetic improvement is to increase profitability, and the FCE of the herd is an important component of this because feed costs are an important part of the cost of beef production. Herd FCE can be increased by increasing net reproductive rate, by increasing growth rate without increasing cow maintenance requirement or by decreasing NFI. NFI is heritable and responds to selection but it is so expensive to measure that few stud breeders routinely measure it. The most likely method of selection in the near future is genomic selection based on a dense panel of SNP markers. There are many physiological processes that contribute to variation in NFI and these are controlled by hundreds, if not thousands, of genes, each with a small effect on NFI. Despite this physiological complexity, genomic selection can result in cattle with reduced NFI and overall greater profitability and is likely to be widely implemented in years to come.
August 2011